Designing Machine Learning Systems
Автор: Chip Huyen (2022)
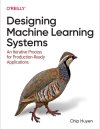
Ever since the first machine learning course I taught at Stanford in 2017, many people have asked me for advice on how to deploy ML models at their organizations. These questions can be generic, such as “What model should I use?” “How often should I retrain my model?” “How can I detect data distribution shifts?” “How do I ensure that the features used during training are consistent with the features used during inference?” These questions can also be specific, such as “I’m convinced that switching from batch prediction to online prediction will give our model a performance boost, but how do I convince my manager to let me do so?” or “I’m the most senior data scientist at my company and I’ve recently been tasked with setting up our first machine learning platform; where do I start?” My short answer to all these questions is always: “It depends.” My long answers often involve hours of discussion to understand where the questioner comes from, what they’re actually trying to achieve, and the pros and cons of different approaches for their specific use case. ML systems are both complex and unique. They are complex because they consist of many different components (ML algorithms, data, business logic, evaluation metrics, underlying infrastructure, etc.) and involve many different stakeholders (data scientists, ML engineers, business leaders, users, even society at large). ML systems are unique because they are data dependent, and data varies wildly from one use case to the next. For example, two companies might be in the same domain (ecommerce) and have the same problem that they want ML to solve (recommender system), but their resulting ML systems can have different model architecture, use different sets of features, be evaluated on different metrics, and bring different returns on investment. Many blog posts and tutorials on ML production focus on answering one specific question. While the focus helps get the point across, they can create the impression that it’s possible to consider each of these questions in isolation. In reality, changes in one component will likely affect other components. Therefore, it’s necessary to consider the system as a whole while attempting to make any design decision. This book takes a holistic approach to ML systems. It takes into account different components of the system and the objectives of different stakeholders involved. The content in this book is illustrated using actual case studies, many of which I’ve personally worked on, backed by ample references, and reviewed by ML practitioners in both academia and industry. Sections that require in-depth knowledge of a certain topic—e.g., batch processing versus stream processing, infrastructure for storage and compute, and responsible AI—are further reviewed by experts whose work focuses on that one topic. In other words, this book is an attempt to give nuanced answers to the aforementioned questions and more. When I first wrote the lecture notes that laid the foundation for this book, I thought I wrote them for my students to prepare them for the demands of their future jobs as data scientists and ML engineers. However, I soon realized that I also learned tremendously through the process. The initial drafts I shared with early readers sparked many conversations that tested my assumptions, forced me to consider different perspectives, and introduced me to new problems and new approaches. I hope that this learning process will continue for me now that the book is in your hand, as you have experiences and perspectives that are unique to you. Please feel free to share with me any feedback you might have for this book, via the MLOps Discord server that I run (where you can also find other readers of this book), Twitter, LinkedIn, or other channels that you can find on my website
Автор: Chip Huyen (2022)
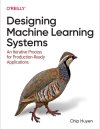
Ever since the first machine learning course I taught at Stanford in 2017, many people have asked me for advice on how to deploy ML models at their organizations. These questions can be generic, such as “What model should I use?” “How often should I retrain my model?” “How can I detect data distribution shifts?” “How do I ensure that the features used during training are consistent with the features used during inference?” These questions can also be specific, such as “I’m convinced that switching from batch prediction to online prediction will give our model a performance boost, but how do I convince my manager to let me do so?” or “I’m the most senior data scientist at my company and I’ve recently been tasked with setting up our first machine learning platform; where do I start?” My short answer to all these questions is always: “It depends.” My long answers often involve hours of discussion to understand where the questioner comes from, what they’re actually trying to achieve, and the pros and cons of different approaches for their specific use case. ML systems are both complex and unique. They are complex because they consist of many different components (ML algorithms, data, business logic, evaluation metrics, underlying infrastructure, etc.) and involve many different stakeholders (data scientists, ML engineers, business leaders, users, even society at large). ML systems are unique because they are data dependent, and data varies wildly from one use case to the next. For example, two companies might be in the same domain (ecommerce) and have the same problem that they want ML to solve (recommender system), but their resulting ML systems can have different model architecture, use different sets of features, be evaluated on different metrics, and bring different returns on investment. Many blog posts and tutorials on ML production focus on answering one specific question. While the focus helps get the point across, they can create the impression that it’s possible to consider each of these questions in isolation. In reality, changes in one component will likely affect other components. Therefore, it’s necessary to consider the system as a whole while attempting to make any design decision. This book takes a holistic approach to ML systems. It takes into account different components of the system and the objectives of different stakeholders involved. The content in this book is illustrated using actual case studies, many of which I’ve personally worked on, backed by ample references, and reviewed by ML practitioners in both academia and industry. Sections that require in-depth knowledge of a certain topic—e.g., batch processing versus stream processing, infrastructure for storage and compute, and responsible AI—are further reviewed by experts whose work focuses on that one topic. In other words, this book is an attempt to give nuanced answers to the aforementioned questions and more. When I first wrote the lecture notes that laid the foundation for this book, I thought I wrote them for my students to prepare them for the demands of their future jobs as data scientists and ML engineers. However, I soon realized that I also learned tremendously through the process. The initial drafts I shared with early readers sparked many conversations that tested my assumptions, forced me to consider different perspectives, and introduced me to new problems and new approaches. I hope that this learning process will continue for me now that the book is in your hand, as you have experiences and perspectives that are unique to you. Please feel free to share with me any feedback you might have for this book, via the MLOps Discord server that I run (where you can also find other readers of this book), Twitter, LinkedIn, or other channels that you can find on my website
Скрытое содержимое могут видеть только пользователи групп(ы): Premium, Местный, Свои